Allgemeine Informationen zu unseren Fallstudien (Case Studies)
Die hier vorgestellten Case Studies stellen Referenzbeispiele für die Anwendung der Sozialen Netzwerkanalyse in der Organisationspraxis dar und dienen den Zielen
- die Anwendung und den Nutzen der Sozialen Netzwerkanalyse zur Identifizierung, Analyse und Visualisierung der Strukturen, Inhalte und Wissensflüsse von informellen persönlichen Netzwerken zu zeigen, die innerhalb und zwischen Organisationen existieren;
- die Ansatzmöglichkeiten für Interventionen und Folgeaktivitäten aufzuzeigen, die auf Basis der Sozialen Netzerkanalyse abgeleitet werden können, um die Prozesse der Wissenskommunikation im Sinne de Organisationziele zu unterstützen und zu fördern.
Alle Case Studies wurden vom Autor dieser Seiten durchgeführt und können in dem hier vorgestellten Buch im Volltext nachgelesen werden (Kapitel 5); Buch und Fallstudien sind nur auf Englisch verfügbar; Copyright: Tobias Müller-Prothmann; Nutzung und Nachdruck nur mit ausdrücklicher schriftlicher Genehmigung des Autors – für entsprechende Anfragen nutzen Sie bitte unser Kontaktformular.
About the Case Study “Leveraging Organizational Expertise”
Identification, development, and conservation of expertise are critical to organizational success or failure. Social network analysis provides a tool to identify expertise within organizations. Reasons to undertake a network analysis for the identification of expertise can be, for example:
- evaluation of quantity and quality of existing expertise or development of new expertise due to strategic reasons,
- lack of experts and expertise,
- lack of transparency of expertise,
- barriers in the communication of expertise,
- poor conservation of existing expertise,
- overload of individual experts.
This case study was undertaken as a small part of the project “Wachstum mit Wissen” (economic growth through knowledge), sponsored by the German Federal Ministry of Education and Research (BMBF), at the Fraunhofer Institute for Production Systems and Design Technology (Fraunhofer IPK), Berlin, in co-operation with the author at the Department of Information Science, Freie Universität Berlin. Names have been changed at the request of the company; selected visualizations have been simplified for presentation.
Preliminary results of this case study were presented and discussed at the Sunbelt 2004, XXIV. International Social Network Conference, May 12-16, 2004, Portoroz / Slovenia (see Müller-Prothmann 2004) and at the I-KNOW 04, 4th International Conference on Knowledge Management, June 30-July 2, 2004, Graz / Austria (see Müller-Prothmann and Finke 2004b, also published as Müller-Prothmann and Finke 2004a) (see Publications).
Goal
The case study has been undertaken in a research and service organization to examine its expertise within the domain of acquisition of EU fundings for research projects. To illustrate this example, the purpose of this case study can be outlined briefly as follows:
Consider that you are working in a research and service organization. You have to acquire funding for new research projects and you know that programs and financing are available from the European Union (EU). You have an idea for a new project, but you do not know how best to prepare a project proposal. However, there are other people in your organization who have successfully acquired EU funding for their projects. The question is: How do you find out who knows about developing a winning proposal for an EU project acquisition? Who are the experts in your organization and who do you need to know and contact outside of your organization to assist you (for instance, at the European Commission)?
Basic conditions
The case study exemplified here aims at the identification of expertise with regard to knowledge about the acquisition of EU fundings for research projects. This domain of knowledge was differentiated into five sub-domains that are important for success or failure of the acquisition of EU funding. These basic sub-domains were derived through methods of integrated enterprise modeling (see Spur et al. 1996; Mertins and Jochem 1997) and validated with process experts. The relevant sub-domains that were identified are:
- knowledge of the acquisition process,
- knowledge of finished or ongoing projects,
- knowledge of potential partners,
- knowledge of funding topics,
- knowledge of key people in the relevant EU institutions.
Other basic conditions were derived from the assumptions of factors that facilitate or hinder knowledge communication between people in personal networks. Factors that have been studied were
Factors that facilitate knowledge transfer:
- experience,
- specialized knowledge and competence,
- continuous exchange of knowledge,
- spatial proximity,
- mutual understanding,
- mutual completion.
Barriers that hinder knowledge transfer:
- hierarchies,
- lack of time and availability,
- organizational boundaries.
In addition, it was taken into account that people make use of the possibility to avoid existing barriers. This is especially relevant for the case that people contact experts who are not easily available to them through the intermediation of third persons who are easier to contact.
Method
A questionnaire was mailed to collect the data for the social network analysis about expertise of EU funding. The questionnaire was used to identify the experts according to the relevant sub-domains outlined above. This was reached through a combination of self-assessment of the participants, through assessment by others, and through results of the network analysis (see figure). Besides, integration and availability of expert knowledge was analyzed. Barriers and factors to facilitate the contacts between the network members were explored with regard to the different sub-domains.
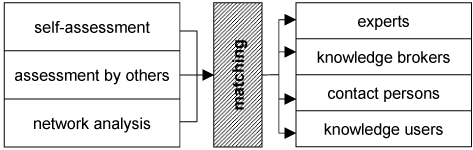
Case Study: Matching Method for Expert Identification (Source: Müller-Prothmann, T. "Leveraging Knowledge Communication for Innovation", 2006)
The identification of potential networks with regard to the central position of experts, compared with the existing network of central contact persons, allows insights into
- knowledge transfer between experts and non-experts,
- knowledge transfer between experts,
- popularity of experts across organizational boundaries,
- boundary-spanning knowledge transfer.
The survey was held in November 2002. All research staff of the organization was invited to participate. A total of 25 people finally participated in the survey which is equal to a participation rate of 28 per cent.
Selected Results
This case study lead to a set of important findings, some of the most important are presented here. In this section, the main findings are presented with regard to (1) size of the network, (2) number of experts, (3) seniority, (4) self-assessment of the participants, and (5) barriers and enablers. In addition, visualizations and analysis of the results are presented with regard to the different sub-domains concerning (1) knowledge of the acquisition process, (2) knowledge of finished or ongoing projects, (3) knowledge of potential partners, (4) knowledge of domains of ongoing fundings, and (5) knowledge of key people in the relevant EU institutions.
- Network size: The network analyzed in this study has 42 members (actors). Although the data is based on the answers of 25 participants only, people could name other persons as experts or contacts who did not submit a questionnaire themselves. Therefore, the network size is larger than the number of answered questionnaires. 86 per cent of the network members are part of the research staff (in the fields of automation and robotic, virtual product development, enterprise management) or administration / management staff of the organization, and 14 per cent are external people (members of the corporate administration or partners in joint projects).
- Number of experts: Different people were named as experts with regard to the different sub-domains. All sub-domains aggregated, 10 people could be identified as experts by four or more participants. With regard to the different sub-domains, only three people could be identified as experts named by four or more participants. In the following sections, only those network members are called experts who were named by four or more people.
- Number of contact persons: As for the experts, those people are called contact persons who were identified as contact persons by four or more participants. Thus, a total of six people were identified as contact persons according to this criterion.
- Seniority: About 50 per cent of the network actors were members of the organization for four years or more. The figure below shows the seniority distribution according to the percentage share between experts and non-experts. The higher seniority of experts is obvious.
- Self-assessment of the participants: Self-assessment of the participants shows that experts rank their own expertise as relatively high. The self-assessment of non-experts matches the assessment by the others. This leads to the conclusion that the perception of the participants of themselves corresponds positively with the perception by others.
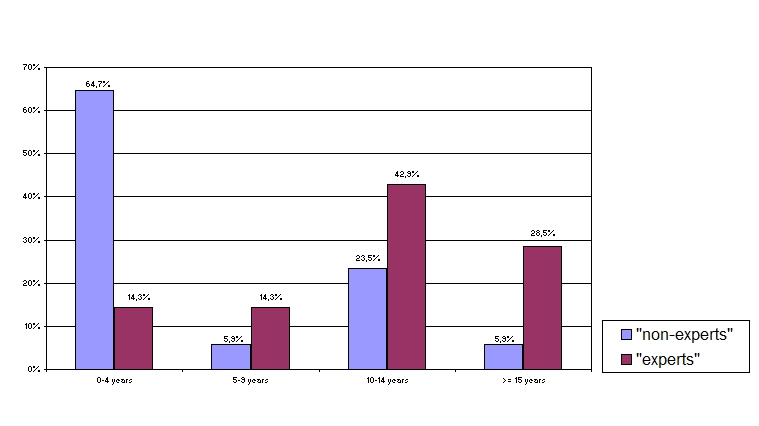
Case Study: Seniority Distribution Between Experts and Non-Experts (Source: Müller-Prothmann, T. "Leveraging Knowledge Communication for Innovation", 2006)
Visualizations of the results and key findings of the network analysis are presented with regard to the different sub-domains: (1) knowledge of the acquisition process, (2) knowledge of finished or ongoing projects, (3) knowledge of potential partners, (4) knowledge of funding topics, and (5) knowledge of key people in the relevant EU institutions. Coloring and grouping of the nodes, which represent the network members, indicate their membership to organizational sub-units. The directed lines indicate who has been named an expert or contact person by whom. The strength of lines indicates the priority of expertise as named by the participants. Experts are indicated by an “E”, contact persons by an “A”. Here we present only two selected results with regard to the two sub-domains (1) Knowledge of the acquisition process and (5) Knowledge of external key persons in the relevant EU institutions.
Knowledge of the acquisition process: The figure below shows the relationships of expert identifications with regard to knowledge of the acquisition process of EU projects (“Whom do you consider as an expert with regard to successful acquisition of EU projects?”). The next figure below shows the corresponding relationships of contact persons as identified in the study (“Whom do you contact for expertise about the acquisition of EU projects?”). One expert could be identified according to the definition criterion given above (four people or more consider him or her an expert). Considering all expert-relationships, 13 participants mentioned experts from their own organizational unit, while 8 were mentioned across organizational boundaries. The ratio between internal and external ties (we could call this the “intra-/inter-ratio”), i.e. the number of identified experts from the own versus the number of identified experts from other organizational units, has a value of 1.625. To compare configurations of internal and external linkages, Krackhardt and Stern (1988) introduced the E-I index as a normalized measure of the ratio between external and internal relationships. The E-I index measures the ratios between external and internal ties and normalizes them to a value within the range of -1.0 to +1.0. An E-I index of -1.0 would indicate that only internal relationships exist, while all relationships would be external for an E-I index of +1.0. Here, the E-I index reaches a score of -0.2381. The expert identified in figure ‘Experts Project Acquisition’ is also a contact person (see figure ‘Contact Persons Project Acquisition’). In addition, one more contact person was identified. The cluster in the lower right is characterized through internal relationships of contacts only. Moreover, it is remarkable that 36 contact relationships are internally established within organizational units, while inter-organizational relationships exist only for 10 relations (E-I index = -0.5652). We can conclude with regard to knowledge of the acquisition process that knowledge of the experts is requested by others, but requests mainly remain internal to the
corresponding organizational sub-unit.
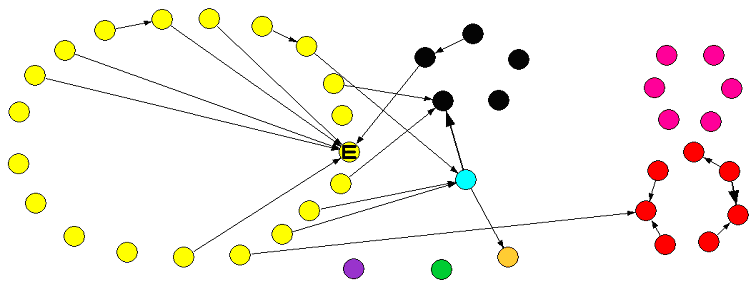
Case Study: Experts Project Acquisition (Source: Müller-Prothmann, T. "Leveraging Knowledge Communication for Innovation", 2006)
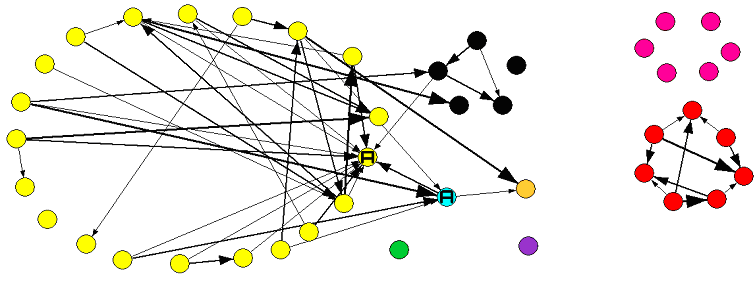
Case Study: Contact Persons Project Acquisition (Source: Müller-Prothmann, T. "Leveraging Knowledge Communication for Innovation", 2006)
Knowledge of external key persons in the relevant EU institutions: The figure below shows the expert identifications of people who have knowledge of and relationships with key persons from relevant EU institutions (“Whom do you consider as an expert with regard to connections to key persons from relevant EU institutions?”). The next figure below shows the corresponding relationships of contact persons as identified in the study (“Whom do you contact for information about key persons from EU institutions?”). One person could be identified as having informations about and connections to external key people from relevant EU institutions. The E-I index is well-balanced (0.0000). But it is obvious that only a small number of people is named as expert again. Also, the identified expert is contacted only by two people from his or her own organizational unit. The identified contact person is exclusively contacted from his or her own organizational unit as well. The E-I index of identified contact relationships is -0.5000.
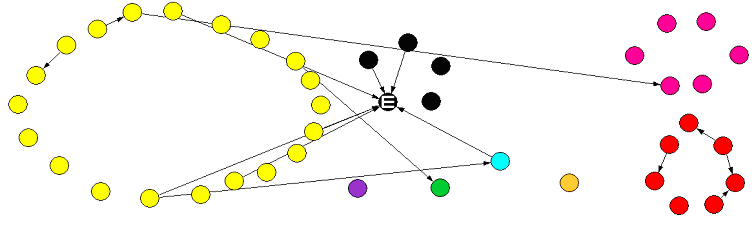
Case Study: Experts External Key Persons (Source: Müller-Prothmann, T. "Leveraging Knowledge Communication for Innovation", 2006)
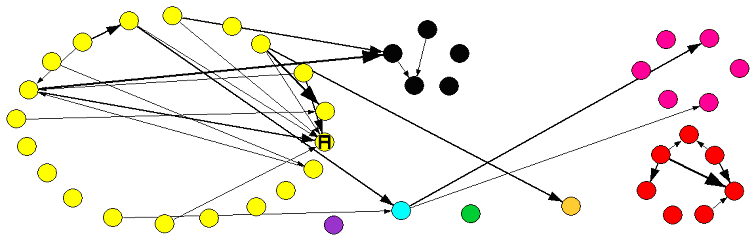
Case Study: Contact Persons External Key Persons (Source: Müller-Prothmann, T. "Leveraging Knowledge Communication for Innovation", 2006)
The results impressively show that not the people with the best expertise are contacted but that other people play the role of contact persons. Moreover, contacting people strongly depends on organizational membership. The average E-I index for experts (i.e. the normalized measure of the ratio between the number of identified experts from the own versus the number of identified experts from other organizational units) is -0.2432 (std. dev.: 0.1481) whereas the average E-I index for contact persons (i.e. the normalized measure of the ratio between the number of identified contact persons from the own versus the number of identified contact persons from other organizational units) is -0.4993 (std. dev.: 0.1810).
This leads to the conclusion that people know about existing expertise throughout the organization, but when they need to actually contact people, they address members of their own organizational unit. This gives reason to assume the existence of other factors besides expertise which influence the informal flows of knowledge. While organizational barriers (i.e. membership within different organizational units) have not been mentioned by the participants as answers to the direct question about existing barriers, network analysis clearly indicates the influence of organizational membership on informal relationships of knowledge flows, particularly on contacting people. Moreover, other barriers than organizational membership seem to be relevant as well, as the position of the expert in the third and fifth sub-domain of knowledge suggests. Due to the differences between results from direct answers about barriers and results from the actual network, the suspicion arises that factors of social acceptance have influence on the answers given by the participants, i.e. the persons questioned are supposed to give answers that seem to be socially wanted for the case of direct questions. These differences could be revealed through the results of social network analysis.
Lessons Learned
As mentioned above, only a total of 25 people finally participated in the survey which is equal to a participation rate of 28 per cent. Although this return rate is at a common level, network analysis aims at reaching a return rate of at least 66 per cent or, better, even more. The low return rate in this case study is a result primarily due to two reasons:
- although all data was anonymized for the analysis, network data collection is always person-related—therefore, social network analysis in organizations is always very sensitive to privacy issues;
- a majority of the research staff was never involved in processes of project acquisition—therefore, these people did not feel addressed to participate in the study.
Although the low return rate reduces the validity of an extensive interpretation of the results, the primary goal of this pre-test study was reached: to pragmatically adapt the method of social network analysis for organizational practice, to gain experience, and to provide explorative explanation of its use and limits. The results of this case study have been documented and have been the foundation to develop a guideline to apply social network analysis as an analytical knowledge management method in other business units of the organization as well.
From a methodical point of view, the questionnaire design was well elaborated, but did miss to check validity of the answers with regard to one aspect: reciprocity. Network linkages in this case study are directed, but the questionnaire collected only data for one direction only (“Whom do you consider as an expert with regard to…?”) and did not ask for the reciprocal connection (like, e.g., “Who did assist you with his or her expertise with regard to…?” and “Whom did you assist with your expertise with regard to…?”). Despite this methodical weakness of the survey design, the workshop discussions finally indicated the correctness and usefulness of the results anyway.
To conclude, this example shows that social network analysis provides a method to trace knowledge flows, analyze network structures and personal expertise with additional value to a simple knowledge map or yellow pages. On the one hand, social network analysis tries to identify knowledge flows wherever they may go. On the other hand, social network analysis gives us a detailed picture of actors’ positions, the characteristics of their connections and the overall structure of relationships. Social network analysis provides us with a well-elaborated set of methods and measures for various applications in knowledge management that especially help us to foster organizational expertise and transfer of knowledge.